Data dodania: 2023-12-11
Seminarium Wydziału Fizyki Technicznej i Matematyki Stosowanej 15.12. o 11:15
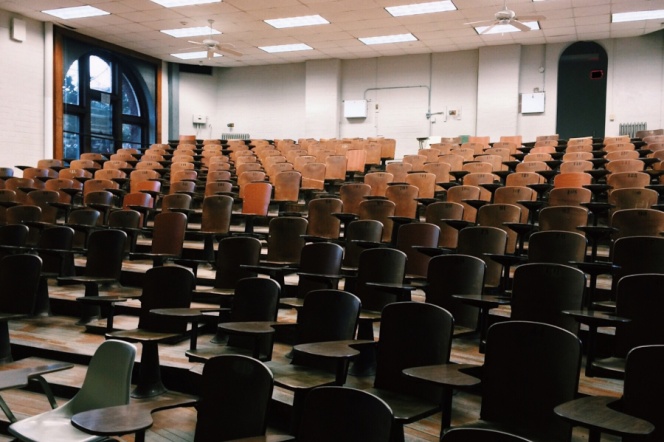
Szanowni Państwo,
informujemy, że w dniu 15 grudnia (piątek) o godzinie 11:15 w sali 121 w Gmachu Głównym
Hiromichi Suetani
Faculty of Science and Technology, Oita University, Japan and International Research Center for Neurointelligence (IRCN), The University of Tokyo, Japan
wygłosi wykład pt.: "Exploration of human individuality in macroscopic brain dynamics through manifold learning"
Abstract: The question of how much individuality is reflected in macroscopic brain dynamics has long been of interest, along with individuality in fingerprints, faces, or gait patterns [1]. For example, Vogel et al. explored the direct correlation between the morphology of EEG patterns and an individual's genotype by comparing the similarity of EEG recordings between identical and fraternal twins. They reported that certain EEG characteristics, particularly occipital alpha power and peak frequency, are highly heritable [2].
In this presentation, we first present our recent work on visualizing individuality and interindividual differences in macroscopic human brain dynamics in low-dimensional space using manifold learning techniques [3]. We propose an appropriate dissimilarity measure between multichannel EEG signals using a unified divergence measure between power spectra known as beta-divergence [4]. We then combine standard manifold learning methods such as ISOMAP, t-SNE, and UMAP with a dissimilarity measure based on beta divergence and apply it to resting-state EEG signals obtained from 100 healthy participants. The results show that each participant is represented as an isolated point cloud in a low-dimensional space, highlighting inter-individual variability and relationships between point clouds.
In addition to the linear correlation (power spectrum) approach described above, we also present results of differences in macroscopic brain dynamics between healthy controls and schizophrenic patients using a dissimilarity measure based on phase synchronization between EEG time series measured at each electrode. Furthermore, as an attempt using nonlinear time series modeling, we also show an approach based on reservoir computing approach [5].
[1] M. DelPozo-Banos, C.M. Travieso, C.T. Weidemann, and J.B. Alonso, EEG biometric identification: a thorough exploration of the time-frequency domain. J. Neural Eng. 12 (5), 056019 (23 pages) (2015).
[2] F. Vogel, Genetics and the Electroencephalogram. Springer (2000).
[3] H. Suetani and K. Kitajo, A manifold learning approach to mapping individuality of human brain oscillations through beta-divergence. Neuroscience Research 156 188–196 (2020).
[4] A. Cichocki, R. Zdunek, S.I. Amari, Families of alpha-beta-and gamma-
divergences: flexible and robust measures of similarities. Entropy 12 (6), 1532–1568 (2010).
[5] H. Suetani and K. Kitajo, Exploring Individuality in human EEG using reservoir computing. In: International Conference on Artificial Neural Networks. Cham: Springer Nature Switzerland, 551-555 (2023).
-
2024-04-22
Program mentoringowy IT for SHE